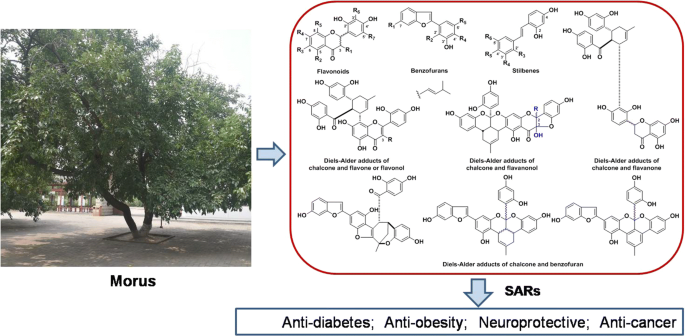
The Role of Structure-Activity Relationships and Expert Systems
In vitro human skin permeability coefficient data collected by Flynn (1990) have been analysed using multiple regression analysis.
An improved model for the prediction of permeability coefficients has been derived by the inclusion of the melting point as an independent variable in addition to the octanol-water partition coefficient (as logP) and molecular volume.
(The solubility of chemicals in water is related to logP and melting point through an algorithm; Suzuki, Journal of Computer-Aided Molecular Design 1991, 5, 149-166).
Examination of the dataset using principal components analysis confirmed that it could be divided into three distinct groups of chemicals-steroids, other pharmacologically active molecules.
A sub-set of hydrocortisone derivatives within the steroid group had measured permeability coefficients which were around 1.5 orders of magnitude greater than values predicted from the small molecular model.
By grouping together the small molecules and the steroids (excluding the hydrocortisone set)-60 molecules-a quantitative structure-activity relationship (QSAR) has been derived for their permeability coefficients which is dependent on logP, molecular volume and melting point, and which explains more than 90% of the variability in the data.
Although yet to be tested experimentally, this QSAR is expected to give accurate predictions of in vitro human skin permeability coefficients within the constraints of the dataset.
A historical database containing the results of 294 defined single substances tested in the guinea-pig maximisation test, carried out according to a single protocol, was used to derive a set of structural alerts for skin sensitisation, which have been incorporated into the expert system, DEREK.
Together with an assessment of percutaneous absorption, this system forms an integral part of a strategic approach to the identification of contact allergens.
Quantitative structure-activity relationships (QSARs) were derived for the skin corrosivity of organic acids and bases, and for the eye irritation potential of neutral organic chemicals.
The independent variables used for these analyses were selected on the basis of the putative mechanisms for skin irritation or corrosivity and for eye irritation, respectively.
Data sets were analysed using principal components analysis; plots of the first two principal components for different classifications of toxicological activity. The derived QSARs are expected to give useful predictions of skin corrosivity and eye irritancy for new or untested chemicals in these classes.
Although the development of these techniques is still at a very early stage, they are already able to play an important part in proposed strategies for the reduction of experimental animal usage.
In the long term, it should be possible to conduct safety evaluations using fewer experimental animals or no animals at all.
However, acceptance by regulatory authorities will be a key factor in realising the full benefits of the approach.
Quantitative structure-activity relationships (QSARs) have been derived by relating skin irritation and corrosivity data of neutral and electrophilic organic chemicals to their log(octanol/water partition coefficient) (logP), molecular volume, dipole moment and 1/molecular weight. Datasets were analysed using stepwise regression, discriminant and principal components analysis.
Discriminant analysis between irritant and non-irritant neutral and electrophilic organic chemicals using the above parameters, which broadly model skin permeability (logP and molecular volume), ‘reactivity’ (dipole moment) and 1/molecular weight to compensate for the fact that skin irritation/corrosivity testing is carried out using a fixed mass or volume of chemical, was found to discriminate well for only 73.1% of the dataset (67.3% cross-validated).
The poor discrimination at the irritant/non-irritant classification boundary is attributed largely to biological variability. Stepwise regression analysis of the Primary Irritation Index (PII) for the same dataset showed a poor correlation (r2=0.422; cross-validated r2=0.201) with a positive dependence on logP and dipole moment and a negative dependence on molecular volume, 1/molecular weight was not a significant variable.
While this QSAR for PII has little value as a predictive model, mainly because of the large biological variability evident in PII values, it is useful in confirming the putative model for skin irritation.
Discriminant analysis using logP, molecular volume and dipole moment, was able to dicriminate reasonably well (92.9% well classified; 92.9% cross-validated) between corrosive and non-corrosive electrophiles.
A plot of the first two principal components of the same parameters showed a clear demarcation between corrosive and non-corrosive electrophiles.
In contrast to the QSARs for skin irritation, increasing skin corrosivity was found to correlate with decreasing molecular olume, with incresing dipole moment, and with decreasing logP.
The predominant parameter in determining the skin corrosivity of electrophilic organic chemicals appears to be the molar dose at which they are tested; this arises because skin corrosivity testing is conducted using a fixed mass or volume of chemical.
A stepwise approach to the skin corrosivity/irritation classification of neutral and electrophilic organic chemicals is outlined. The derived QSARs should be useful for the prediction of the skin corrosivity potential of new or untested electrophiles. (Non-electrophilic neutral organic chemicals, as a category, do not generally appear to be corrosive).
Discrimination between some non-irritant and irritant neutral and electrophilic organic chemicals using these techniques is also possible.
The Role of Structure-Activity Relationships and Expert SystemsFor a large number of chemicals whose irritation potentials lie in a fairly broad and around the irritant/non-irritant, classifiction boundary, no firm prediction of classification is possible.